To visualize a decision tree in scikit-learn, you can use the plot_tree
function from the sklearn.tree
module. This function allows you to generate a visual representation of the decision tree.
Here’s a simple example:
from sklearn import tree
plt.figure(figsize=(20,30))
tree.plot_tree(model, feature_names=feature_names, filled=True, fontsize=9, node_ids=True, class_names=True)
plt.show()
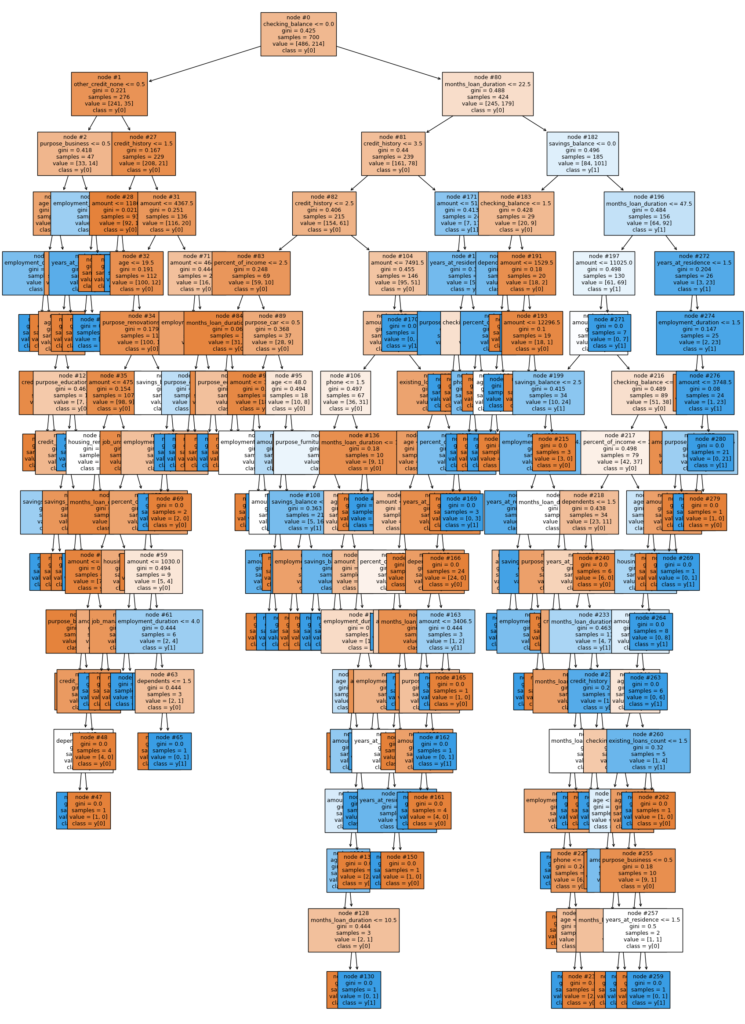
To show the decision tree as text in scikit-learn, you can use the export_text
function from the sklearn.tree
module. This function generates a textual representation of the decision tree.
Here’s an example:
from sklearn.tree import DecisionTreeClassifier, export_text
from sklearn.model_selection import train_test_split
# Create a decision tree classifier (you can replace this with your own model)
clf = DecisionTreeClassifier()
# Assuming X_train and y_train are your training data
clf.fit(X_train, y_train)
# Generate a text representation of the decision tree
tree_text = export_text(clf, feature_names=list(X_train.columns), show_weights=True)
# Print the text representation
print(tree_text)
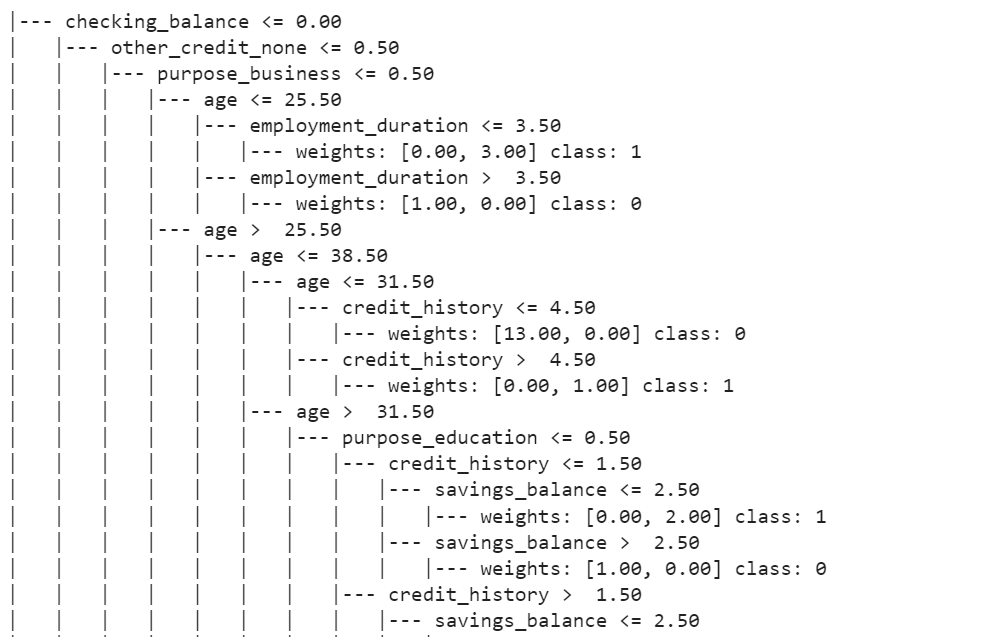