StandardScaler
is a preprocessing technique used to standardize features by removing the mean and scaling them to have a unit variance. Standardization is a common step in many machine learning algorithms, especially those that involve distance-based calculations or optimization processes, as it helps ensure that all features contribute equally to the analysis.
fit_transform
computes the mean and standard deviation of each feature in data
and then scales and centers the data based on these statistics.
Here’s a brief overview of how to use the StandardScaler
in scikit-learn:
# standardizing the data
from sklearn.preprocessing import StandardScaler
scaler = StandardScaler()
data_scaled = scaler.fit_transform(data)
# statistics of scaled data
pd.DataFrame(data_scaled).describe()
Before standardization:
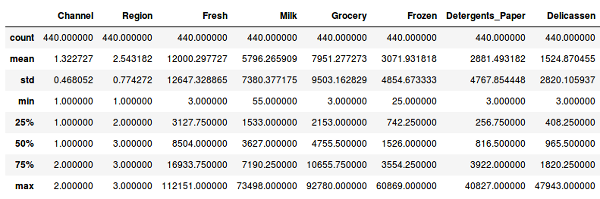
After standardization:
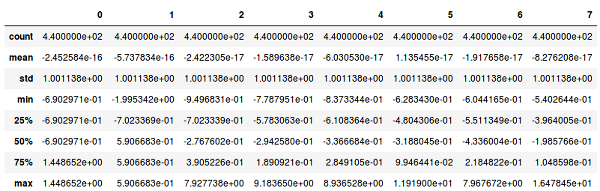